TM© THE E-DITOR'S NOTE
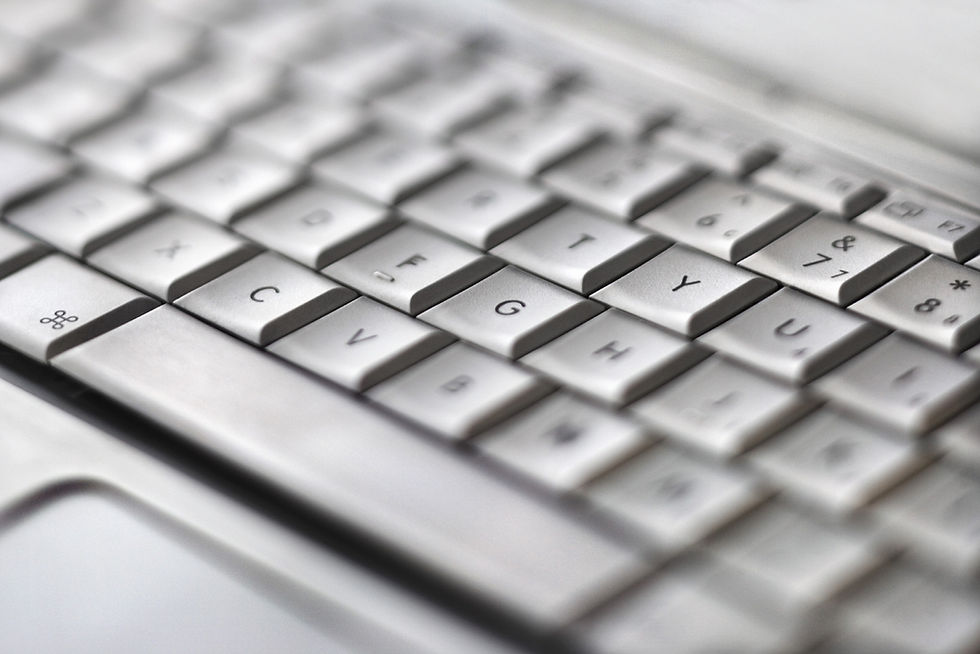
[ARCHIVE] SPOTLIGHT
This is an archived topic. To find and/or revisit any of our past Spotlight topics, visit our Archive.
TECHNOLOGY
SHARE THIS
Generative AI In Supply Chain
Generative AI is the very embodiment of the concept of technological impact at scale and a big breakthrough that delivers on the promise of process optimization, cost and time saving. Generative AI can help revolutionize a multitude of business areas in various industries, with supply chain being one of the most important ones.
Supply chain is an integral part of businesses. Streamlining supply chain operations, can help minimize bottlenecks, reduce delays, costs and risks, and enhance productivity. It can also play a strategic role in helping businesses achieve goals related to customer satisfaction and loyalty. Among the several use cases and potential business benefits of generative AI, here we will explore some of the ways that generative AI can help optimize supply chain operations in some key industries.
Some Key Types Of Supply Chain In Business
-
Supply Chain Product Management - A supply chain is a network of individuals and companies engaged in the process of creating a product and delivering it to the end consumer. This process includes the following key operations: Planning and Demand Management, Procurement, Production Planning, Inventory Management, Transportation, Delivery and Order Fulfillment.
-
Digital Content Supply Chain - A content supply chain is the end-to-end process, workflows, and tools leveraged by businesses to create, manage, and distribute content. This process includes the following key operations: Strategy, Planning and Ideation, Content Development and Production, Quality Review and Publishing Planning, Asset Management, Content Distribution, and Results' Tracking and Reporting.
Defining Supply Chain Bottlenecks
A supply chain bottleneck is a process or phase in supply chain that is preventing the smooth and undisrupted flow in the operational lifecycle. Bottlenecks can be small and cause issues capable of slowing down operations, but they can also turn to chokepoints and grind supply chain to a halt. Supply chain is often faced with bottlenecks that result to a series of delays and inefficiencies and typically occur when process effectiveness falls out of balance. Below are some frequent examples of bottlenecks faced by supply chain.
-
Supply Chain Product Management Bottleneck - In the event in which customer demand exceeds a company's production capacity, then a bottleneck is bound to occur and cause a series of disruptions in the process flow and profitability. Regardless of its magnitude, a bottleneck can cause delays, that have a negative impact on customer satisfaction and loyalty and a brand's reputation, incur additional costs, and result to preventing business operations from running smoothly and effectively.
-
Digital Content Supply Chain Bottleneck - Content is slow to get to market as a vast amount of data needs to be accurately analyzed, then used to generate content that will need to go through various layers of quality control and review, before it can be thoroughly documented and shared with the public. Lack of automation can make any required activities to be cumbersome, which can frequently lead to duplicative effort, while it can turn the process of estimating and/or tracking workforce performance, a real challenge.
Generative AI in Supply Chain Market Size
Global generative AI in supply chain market size was valued at USD 301.83 million in 2022, and is expected to reach approximately USD 12,941.14 million by 2032, growing at a CAGR of 45.62% during the forecast period from 2022 to 2032.
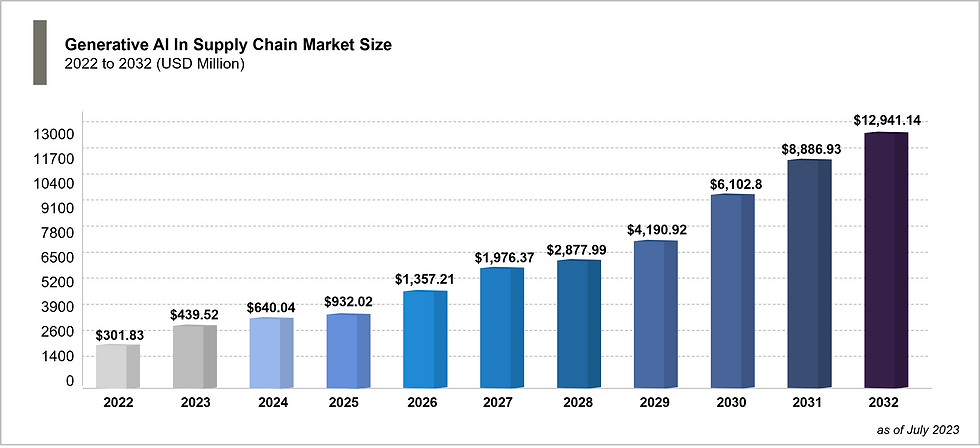
Source: Precedence Research
Generative AI Use Cases in Supply Chain
Below are some indicative use cases of generative AI in supply chain, that can also be the root cause behind the most frequently encountered bottlenecks.
Supply Chain Product Management:
-
Inventory Management - Generative AI can optimize replenishment and give access to real-time and accurate tracking. It can create synthetic data to train machine learning algorithms to help predict demand variations and identify patterns.
-
Demand Forecast – Generative AI can help identify key drivers such as seasonal trends and market shifts. By analyzing historical data, it can identify correlations that companies can use to foresee demand fluctuations with greater accuracy that can help minimize inventory risks.
-
Logistics and Distribution - Generative AI can help update and optimize delivery routes that are typically impacted by volatile factors such as weather and traffic. This can help reduce fuel consumption and improve customer satisfaction.
-
Procurement and Supplier Management – Generative AI can compute real-time pricing benchmarks which combined with the use of historical transactions, can help create tailormade negotiation strategies.
-
Risk Management - Generative AI can enable the automation of manual tasks that typically impact risk management. Through the use of accurate data, risk managers are able to make well-informed decisions and mitigate risk.
Digital Content Supply Chain:
-
Content Production - Generative AI can help with personalization. By leveraging advanced algorithms, it can analyze vast amounts of data, make accurate consumer behavior predictions and automate personalized content creation.
-
Asset Management - Generative AI can provide decision support to asset managers through targeted strategies, asset allocations, automation of marketing tasks, and risk mitigation suggestions based on historical data and market trends.
-
Reporting - Generative AI can be used to analyze vast amounts of unstructured data, that have in the past, been difficult to capture, and extract actionable insights that can be used to make well-informed and strategic decision making.
Examples Of Industries Impacted By Generative AI
FMCG and Pharmaceutical markets are two sectors, within the broader consumer goods industry, that can be benefited by the implementation of generative AI. Each of them presents some unique regulatory requirements, aimed to address different consumer needs, with their distinct characteristics directly affecting the way they are developed, advertised and sold. Let’s take a look at the ways generative AI can help each of the said markets:
FMCG Market - Effective supply chain management is an area of great prominence for Fast-Moving Consumer Goods (FMCG) companies. Why? Because fast moving consumer goods come in high demand, have low-profit margins, while their life expectancy on the shelf is notably brief.
FMCG Market's Investment In Generative AI
Generative AI in FMCG market size is expected to reach a notable growth, as it is estimated to reach a value of USD 57.7 billion by 2033, marking a prominent increase from USD 7.9 billion in 2023, and growing at a CAGR of 22% during the forecast period from 2024 to 2033.
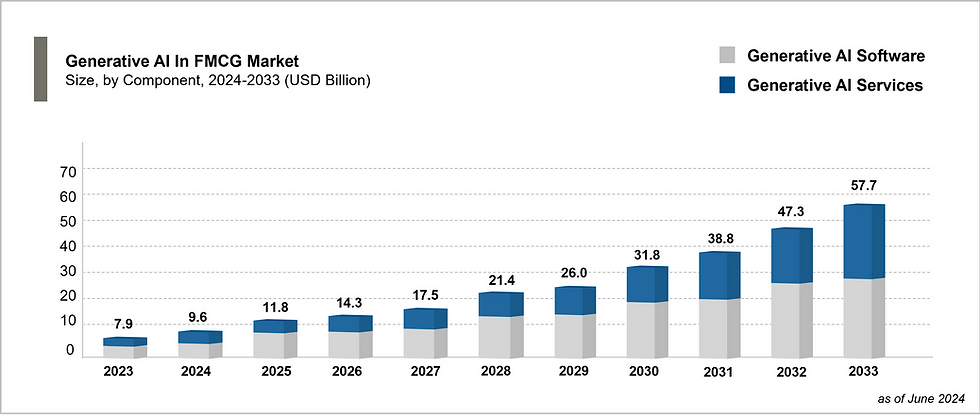
Source: market.us
Pharmaceutical Market - Effective supply chain management is an area of great prominence for pharmaceutical companies. Why? It is actually a twofold; generative AI can optimize operations related to the flow and management of goods while it can help streamline activities that pertain to marketing.
Pharmaceutical Market's Investment In Generative AI
Global Generative AI in pharmaceutical market size is expected to be worth approximately USD 2,258.1 million by 2033 from USD 159.9 million in 2023, growing at a CAGR of 31.2% during the forecast period from 2024 to 2033.

Source: MarketResearch.biz
Example Of Leading Companies In The FMCG Industry Using Generative AI In Supply Chain Product Management
Unilever - Unilever, a global leader in the FMCG market, has a robust manufacturing and distribution system, that serves approximately 3.4 billion consumers worldwide through a global supplier base. In an effort to deliver its trusted consumers efficiently, it is currently integrating generative AI within its supply chain. The use of generative AI and data models is aimed at helping the company stay up to date with the continuous advancement of technology to ensure it drives growth and innovation, but also help minimize its environmental impact to deliver on the promise of sustainability. To tangibly act on its commitments, the company launched its first and only global AI lab in Toronto, Canada, in November 2023.
''Unilever is making a significant investment in developing and deploying AI technology across the business. The launch of Unilever’s first AI lab will expedite the progress we are making globally and enable us to have the right focus on some of the big questions we want to answer with AI''
Andy Hill, Chief Data Officer, Unilever
The company is committed to invest in disruptive innovation and acceleration of AI adoption with the goal to achieve an immediate impact on key areas such as: forecasting, modelling complex data relationships with graph technology, and generating actionable insights and accurate predictions through generative AI-powered reporting of trends and patterns. This move, is part of the company's broader strategy focused on the acceleration of generative AI-powered concepts and projects that can be scaled across its business globally.
''Although 15 areas of focus have been identified, the lab is initially concentrating on the three that have the greatest potential for making an immediate impact: forecasting, modelling complex data relationships with graph technology, and generating insights on trends, patterns and predictions through Gen AI. They are, first, the impact on currently running initiatives and, second, the long-term potential impact of new technology […] Gen AI is also streamlining the access and retrieval of valuable insights from these sites by swiftly categorizing, summarizing and organizing vast amounts of information''
Gary Bogdani, Unilever Head of Data Science for North America and Canada D&A
Example Of Leading Companies In The Pharmaceutical Industry Using Generative AI In Digital Content Supply Chain
Pfizer - Pfizer, a true pioneer in the pharmaceutical industry, has developed a generative AI platform dubbed 'Charlie' to help improve and streamline content supply chains. 'Charlie', that is rolled out across the organization and used across the company's entire spectrum of brands, is currently helping transform the company's marketing operations and strategies and also achieve greater efficiency and accuracy, through the centralization of content management.
''Biggest trend we're seeing is everything related to Chat GPT. Everyone's talking about it. It’s happening faster than I think anyone thought. Generative AI Chat GPT is the beginning of rethinking personalized experiences. I think personal personalization has always been linked to targeting, but now personalization is really about an experience that you're going to have where you're having conversations and you're talking to something that is going to give you answers and we're just at the very beginning of the generative AI trend. We need to talk a lot more about trust particularly as we move into this world of Generative AI and getting answers to things from computers and machines and getting the permission to share information that makes those answers better. We have to rethink trust and relationship with people. It's more than just saying I accept cookies. The trust button of the future is going to be much different and bigger than I accept your cookies because we'll be living in a world that probably won't have cookies for too long''
Drew Panayiotou, Global Chief Marketing Officer, Pfizer
Pfizer is already leveraging AI capabilities, turning to generative AI technology to speed and smarten the work of clinical development. The company is using AI capabilities to calculate vast amounts of data points without errors, aimed at automating the content management process, and tailoring its content to meet the needs, both from a language as well as a technical stand point, of various audiences.
The company is also looking closely at the future benefits of AI for pharma. Specifically, on ways in which AI software can support with accurate documentation and management of content (tables, reports, etc.) related to a new drug’s lifecycle (spanning from development, testing, manufacturing, prescription to its use). It is also acknowledging the way in which the use of machine-learning software can help improve the quality and speed of regulatory submissions, that can help decrease the time needed to get a drug to market, as well as the way in which it can be used to evaluate vast amounts of data to generate actionable insights to help company developers make well-informed decisions and accurate forecasts.
There is also high interest focused on the way AI software can be trained by human experts to produce highly accurate labeling content for a new drug, but also update it faster and with greater accuracy. This can support with investing time wisely when selecting drugs to pursue based on their effectiveness, and by extent, help expedite their distribution to reach populations world-wide.
''Using data to make faster decisions on a medicine’s potential […] would allow us to re-allocate resources, dollars, and expertise to the next promising candidate faster […] we produce tremendous amounts of information and content that we share with the public, with doctors, with regulators and also internal documents that encapsulate our knowledge
[…] we are working to take the content created across the life cycle of a drug at Pfizer — from early analysis and predictions, lab data, and regulatory documentation, to test results and even the booklet that comes with a box of pills from the pharmacy — and both automate its creation, and ensure that it’s of high quality, using AI capabilities […] natural language processing ensures that correct and consistent terminology is used, reducing errors and misunderstandings […] we also need to analyze a tremendous amount of outside content not produced at Pfizer''
Boris Braylyan, Vice President and Head of Information Management at Pfizer
References/Useful Links
-
Generative AI in Supply Chain Market Size, Precedence Research
-
Generative AI In FMCG Market, market.us
-
Generative AI in Pharmaceutical Market, MarketResearch.biz
-
Global Generative AI in Marketing Market, market.us
-
New AI lab to boost innovation, technology and collaboration, Unilever
-
How AI and digital help us innovate faster and smarter, Unilever
-
Artificial Intelligence: On a mission to Make Clinical Drug Development Faster and Smarter, Pfizer
-
Patient-First Health with Generative AI: Reshaping the Care Experience, WEF
Global Generative AI in Marketing Market By Component
The global generative AI in marketing market is valued at USD 2.4 billion in 2023. The overall demand for generative AI in marketing is expected to develop at an 28.6% CAGR over the forecast period from 2023 to 2033. Global sales of generative AI in marketing is anticipated to reach USD 22.2 billion by 2032.
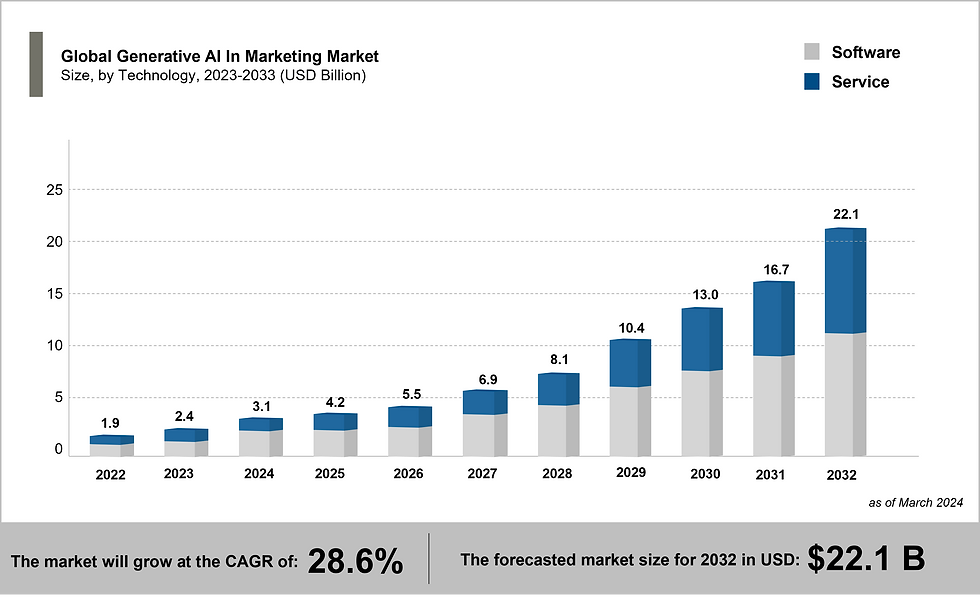
Source: market.us
There is some concern on potential uses of generative AI that are yet to be identified. The landscape of possibilities behind this new and ever-evolving technology seems infinite and yet to be fully explored. However, at its core, generative AI models need to train on human-produced data to function thus are meant to support the human factor and not replace it. It is designed to revolutionize functions and ways of working and add layers of accuracy and security, to help improve the human performance to achieve better quality of service and results.
With that said, the human element will never go from a 'must have' to a 'nice to have' as it is at the epicenter of all the reasons that brought this new technology to existence.
By Eleni Stamoulakatou
June 19, 2024 at 21:00p.m. GR | 1:00p.m. ET
Revised: N/A